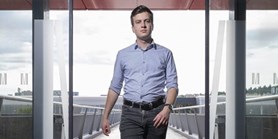
Zde se nacházíte:
Informace o publikaci
Machine Learning in Intrusion Detection: An Operational Perspective
Autoři | |
---|---|
Rok publikování | 2024 |
Druh | Článek ve sborníku |
Fakulta / Pracoviště MU | |
Citace | |
Přiložené soubory | |
Popis | Machine learning has become a prevalent approach in research on intrusion detection with enormous number of research publications on the topic, but its adoption by cybersecurity practitioners is falling behind. Recently, researchers conducted critical and pragmatic assessment of the capabilities of machine learning in this task and identified fundamental issues preventing wider application and easy use in practice. In this paper, we approach the topic from the perspective of network security management, focusing on the issues of compatibility with existing monitoring and security infrastructures, computational complexity, ease of use, and required skills of the operators. The research in machine learning-based intrusion detection strongly favors machine learning metrics (e.g., precision and accuracy) over any other outcome, including performance and usability, for which we have no actual results due to very low number of prototypes, implementations, and field studies. Moreover, there are very limited options of recognizing which type of attack was detected, which remains a strong advantage of traditional signature-based intrusion detection systems. |