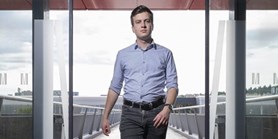
Zde se nacházíte:
Informace o publikaci
Optimizing Rank-based Metrics with Blackbox Differentiation
Autoři | |
---|---|
Rok publikování | 2020 |
Druh | Článek ve sborníku |
Konference | 2020 IEEE/CVF Conference on Computer Vision and Pattern Recognition (CVPR 2020) |
Fakulta / Pracoviště MU | |
Citace | |
www | Springer |
Doi | http://dx.doi.org/10.1109/CVPR42600.2020.00764 |
Popis | Rank-based metrics are some of the most widely used criteria for performance evaluation of computer vision models. Despite years of effort, direct optimization for these metrics remains a challenge due to their non-differentiable and non-decomposable nature. We present an efficient, theoretically sound, and general method for differentiating rank-based metrics with mini-batch gradient descent. In addition, we address optimization instability and sparsity of the supervision signal that both arise from using rank-based metrics as optimization targets. Resulting losses based on recall and Average Precision are applied to image retrieval and object detection tasks. We obtain performance that is competitive with state-of-the-art on standard image retrieval datasets and consistently improve performance of near state-of-the-art object detectors. |