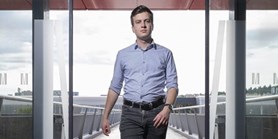
Zde se nacházíte:
Informace o publikaci
Unifying Two Views on Multiple Mean-Payoff Objectives in Markov Decision Processes
Autoři | |
---|---|
Rok publikování | 2015 |
Druh | Článek ve sborníku |
Konference | Thirtieth Annual ACM/IEEE Symposium on Logic in Computer Science (LICS) |
Fakulta / Pracoviště MU | |
Citace | |
Doi | http://dx.doi.org/10.1109/LICS.2015.32 |
Obor | Informatika |
Klíčová slova | Markov decision process; mean payoff; optimization; probability |
Popis | We consider Markov decision processes (MDPs) with multiple limit-average (or mean-payoff) objectives. There exist two different views: (i)~the expectation semantics, where the goal is to optimize the expected mean-payoff objective, and (ii)~the satisfaction semantics, where the goal is to maximize the probability of runs such that the mean-payoff value stays above a given vector. We consider optimization with respect to both objectives at once, thus unifying the existing semantics. Precisely, the goal is to optimize the expectation while ensuring the satisfaction constraint. Our problem captures the notion of optimization with respect to strategies that are risk-averse (i.e., ensure certain probabilistic guarantee). Our main results are as follows: First, we present algorithms for the decision problems, which are always polynomial in the size of the MDP. We also show that an approximation of the Pareto curve can be computed in time polynomial in the size of the MDP, and the approximation factor, but exponential in the number of dimensions. Second, we present a complete characterization of the strategy complexity (in terms of memory bounds and randomization) required to solve our problem. |
Související projekty: |