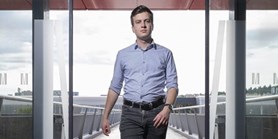
Zde se nacházíte:
Informace o publikaci
Local bandwidth selectors for functional kernel regression
Autoři | |
---|---|
Rok publikování | 2017 |
Druh | Konferenční abstrakty |
Fakulta / Pracoviště MU | |
Citace | |
Popis | Nonparametric regression methods based on kernel smoothing rely on selection of suitable bandwidth parameter in order to minimize the mean squared error. We focus on the functional regression, i.e. the case when the predictor is a functional random variable. In finite-dimensional setting, global selection of bandwidth (same bandwidth is used for all data points) is often sufficient. Due to sparsity of infinite dimensional space, local bandwidth selection (different bandwidth is chosen for each data point) seems to be more suitable approach. In our study, we focus on local bandwidth selection methods. Furthermore, we propose local bandwidth selection method based on penalizing functions. Using simulation studies, we compare local bandwidth selection methods with each other and with their global counterparts. |
Související projekty: |