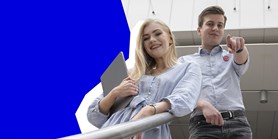
Condensed U-Net (CU-Net): An Improved U-Net Architecture for Cell Segmentation Powered by 4x4 Max-Pooling Layers
Autoři | |
---|---|
Rok publikování | 2020 |
Druh | Článek ve sborníku |
Konference | IEEE 17th International Symposium on Biomedical Imaging |
Fakulta / Pracoviště MU | |
Citace | |
www | https://ieeexplore.ieee.org/abstract/document/9098351 |
Doi | http://dx.doi.org/10.1109/ISBI45749.2020.9098351 |
Klíčová slova | Biomedical Image Segmentation; Convolutional Neural Networks; Deep Learning; Feature Learning; Max Pooling |
Popis | Recently, the U-Net has been the dominant approach in the cell segmentation task in biomedical images due to its success in a wide range of image recognition tasks. However, recent studies did not focus enough on updating the architecture of the U-Net and designing specialized loss functions for bioimage segmentation. We show that the U-Net architecture can achieve more successful results with efficient architectural improvements. We propose a condensed encoder-decoder scheme that employs the 4x4 max-pooling operation and triple convolutional layers. The proposed network architecture is trained using a novel combined loss function specifically designed for bioimage segmentation. On the benchmark datasets from the Cell Tracking Challenge, the experimental results show that the proposed cell segmentation system outperforms the U-Net. |
Související projekty: |