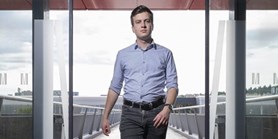
Zde se nacházíte:
Informace o publikaci
FIMSIM: Discovering Communities By Frequent Item-Set Mining and Similarity Search
Autoři | |
---|---|
Rok publikování | 2021 |
Druh | Článek ve sborníku |
Konference | 14th International Conference on Similarity Search and Applications (SISAP) |
Fakulta / Pracoviště MU | |
Citace | |
www | https://link.springer.com/chapter/10.1007%2F978-3-030-89657-7_28 |
Doi | http://dx.doi.org/10.1007/978-3-030-89657-7_28 |
Klíčová slova | community mining;frequent item-set mining;similarity search;network analysis |
Popis | With the growth of structured graph data, the analysis of networks is an important topic. Community mining is one of the main analytical tasks of network analysis. Communities are dense clusters of nodes, possibly containing additional information about a network. In this paper, we present a community-detection approach, called FIMSIM, which is based on principles of frequent item-set mining and similarity search. The frequent item-set mining is used to extract cores of the communities, and a proposed similarity function is applied to discover suitable surroundings of the cores. The proposed approach outperforms the state-of-the-art DB-Link Clustering algorithm while enabling the easier selection of parameters. In addition, possible modifications are proposed to control the resulting communities better. |
Související projekty: |