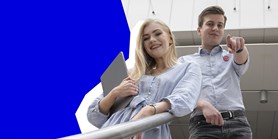
Data-driven Learned Metric Index: an Unsupervised Approach
Autoři | |
---|---|
Rok publikování | 2021 |
Druh | Článek ve sborníku |
Konference | 14th International Conference on Similarity Search and Applications (SISAP 2021) |
Fakulta / Pracoviště MU | |
Citace | |
Doi | http://dx.doi.org/10.1007/978-3-030-89657-7_7 |
Klíčová slova | Index structures; Learned index; Unstructured data; Content-based search; Metric space; Machine learning |
Přiložené soubory | |
Popis | Metric indexes are traditionally used for organizing unstructured or complex data to speed up similarity queries. The most widely-used indexes cluster data or divide space using hyper-planes. While searching, the mutual distances between objects and the metric properties allow for the pruning of branches with irrelevant data -- this is usually implemented by utilizing selected anchor objects called pivots. Recently, we have introduced an alternative to this approach called Lear\-ned Metric Index. In this method, a series of machine learning models substitute decisions performed on pivots -- the query evaluation is then determined by the predictions of these models. This technique relies upon a traditional metric index as a template for its own structure -- this dependence on a pre-existing index and the related overhead is the main drawback of the approach. In this paper, we propose a data-driven variant of the Learned Metric Index, which organizes the data using their descriptors directly, thus eliminating the need for a template. The proposed learned index shows significant gains in performance over its earlier version, as well as the established indexing structure M-index. |
Související projekty: |