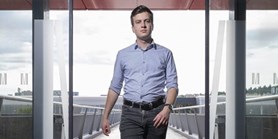
Informace o publikaci
Self-supervised pretraining for transferable quantitative phase image cell segmentation
Autoři | |
---|---|
Rok publikování | 2021 |
Druh | Článek v odborném periodiku |
Časopis / Zdroj | BIOMEDICAL OPTICS EXPRESS |
Fakulta / Pracoviště MU | |
Citace | |
www | https://www.osapublishing.org/boe/fulltext.cfm?uri=boe-12-10-6514&id=459853 |
Doi | http://dx.doi.org/10.1364/BOE.433212 |
Klíčová slova | Self-supervised pretraining; transferable quantitative phase image cell segmentation |
Popis | In this paper, a novel U-Net-based method for robust adherent cell segmentation for quantitative phase microscopy image is designed and optimised. We designed and evaluated four specific post-processing pipelines. To increase the transferability to different cell types, non-deep learning transfer with adjustable parameters is used in the post-processing step. Additionally, we proposed a self-supervised pretraining technique using nonlabelled data, which is trained to reconstruct multiple image distortions and improved the segmentation performance from 0.67 to 0.70 of object-wise intersection over union. Moreover, we publish a new dataset of manually labelled images suitable for this task together with the unlabelled data for self-supervised pretraining. (c) 2021 Optical Society of America under the terms of the OSA Open Access Publishing Agreement |
Související projekty: |