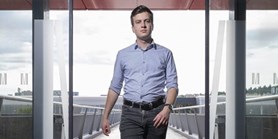
Zde se nacházíte:
Informace o publikaci
Segmentation of Atrial Electrical Activity in Intracardiac Electrograms (IECGs) Using Convolutional Neural Network (CNN) Trained on Small Imbalanced Dataset
Autoři | |
---|---|
Rok publikování | 2021 |
Druh | Článek ve sborníku |
Konference | 2021 Computing in Cardiology (CinC) |
Fakulta / Pracoviště MU | |
Citace | |
www | https://ieeexplore.ieee.org/document/9662729 |
Doi | http://dx.doi.org/10.23919/CinC53138.2021.9662729 |
Klíčová slova | Atrial Electrical Activity; IECGs; CNN) |
Popis | Timing pattern of intracardiac atrial activity recorded by multipolar catheter in the coronary sinus (CS) provides insightful information about the type and approximate origin of common non-complex arrhythmias. Depending on the anatomy of the CS, the atrial activity can be substantially disturbed by ventricular far field complex preventing accurate segmentation by convential methods. In this paper, we present small clinically validated database of 326 surface 12-lead and intracardiac electrograms (ECG and IEGs) and a simple deep learning framework for semantic beat-to-beat segmentation of atrial activity in CS recordings. The model is based on a residual convolutional neural network (CNN) combined with pyramidal upsampling decoder. It is capable to recognize well between atrial and ventricular signals recorded by decapolar CS catheter in multiple arrhythmic scenarios reaching dice score of 0.875 on evaluation dataset. To address a dataset size and imbalance issues, we have adopted several preprocessing and learning techniques with adequate evaluation of its impact on the model performance. |
Související projekty: |