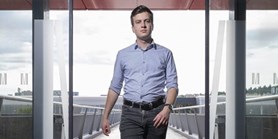
Zde se nacházíte:
Informace o publikaci
Structural MRI-Based Schizophrenia Classification Using Autoencoders and 3D Convolutional Neural Networks in Combination with Various Pre-Processing Techniques
Autoři | |
---|---|
Rok publikování | 2022 |
Druh | Článek v odborném periodiku |
Časopis / Zdroj | Brain Sciences |
Fakulta / Pracoviště MU | |
Citace | |
www | https://www.mdpi.com/2076-3425/12/5/615 |
Doi | http://dx.doi.org/10.3390/brainsci12050615 |
Klíčová slova | schizophrenia; classification; 3D CNN; autoencoders; voxel-based morphometry; deformation-based morphometry; deep learning |
Popis | Schizophrenia is a severe neuropsychiatric disease whose diagnosis, unfortunately, lacks an objective diagnostic tool supporting a thorough psychiatric examination of the patient. We took advantage of today's computational abilities, structural magnetic resonance imaging, and modern machine learning methods, such as stacked autoencoders (SAE) and 3D convolutional neural networks (3D CNN), to teach them to classify 52 patients with schizophrenia and 52 healthy controls. The main aim of this study was to explore whether complex feature extraction methods can help improve the accuracy of deep learning-based classifiers compared to minimally preprocessed data. Our experiments employed three commonly used preprocessing steps to extract three different feature types. They included voxel-based morphometry, deformation-based morphometry, and simple spatial normalization of brain tissue. In addition to classifier models, features and their combination, other model parameters such as network depth, number of neurons, number of convolutional filters, and input data size were also investigated. Autoencoders were trained on feature pools of 1000 and 5000 voxels selected by Mann-Whitney tests, and 3D CNNs were trained on whole images. The most successful model architecture (autoencoders) achieved the highest average accuracy of 69.62% (sensitivity 68.85%, specificity 70.38%). The results of all experiments were statistically compared (the Mann-Whitney test). In conclusion, SAE outperformed 3D CNN, while preprocessing using VBM helped SAE improve the results. |
Související projekty: |