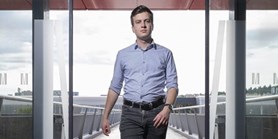
Zde se nacházíte:
Informace o publikaci
Foundation models for the electric power grid
Autoři | |
---|---|
Rok publikování | 2024 |
Druh | Článek v odborném periodiku |
Časopis / Zdroj | Joule |
Fakulta / Pracoviště MU | |
Citace | |
www | https://www.sciencedirect.com/science/article/abs/pii/S2542435124004707 |
Doi | http://dx.doi.org/10.1016/j.joule.2024.11.002 |
Klíčová slova | Foundation Models; Data-Driven Power Grid Modeling; Energy Transition; AI-based Power Flow Simulation |
Popis | Foundation models (FMs), pre-trained on large datasets and adapted to a broad set of applications, are revolutionizing the field of artificial intelligence (AI). Powerful FMs for language and weather have recently emerged, proving that such models can be developed for complex systems. Here, we conceptualize FMs for the electric power grid to be trained on grid data. It is shown that grid FMs have the potential to cope with the increasing complexity and uncertainties stemming from the energy transition by providing a significant speed-up in computation of at least 3–4 orders of magnitude. A key benefit is the generalizability of FMs that enables stakeholders to readily fine-tune the model for specific needs and their own proprietary data in a scalable and economical way. These capabilities make the FM approach ideal for unifying data, technology, and industry expertise toward a common goal. To develop such FM capabilities, we envision the power system and AI communities joining forces in a “moonshot” to develop transformative AI capabilities for the power grid, thereby supporting the energy transition. |