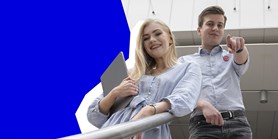
Artificial neural networks combined with experimental design: a “soft” approach for chemical kinetics
Autoři | |
---|---|
Rok publikování | 2012 |
Druh | Článek v odborném periodiku |
Časopis / Zdroj | Talanta |
Fakulta / Pracoviště MU | |
Citace | |
Doi | http://dx.doi.org/10.1016/j.talanta.2012.01.044 |
Obor | Analytická chemie, separace |
Klíčová slova | Chemical kinetics; Soft-modelling; Artificial Neural Networks; Experimental design; Rate constants; Multicomponent analysis; Optimization. |
Popis | The possibilities of artificial neural networks (ANNs) “soft” computing to evaluate chemical kinetic data have been studied. In the first stage, , a set of “standard” kinetic curves with known parameters (rate constants and/or concentrations of the reactants), which is some kind of “normalized maps”, is prepared. The data base should be built according to a suitable experimental design (ED). In the second stage, such data set is then used for ANNs “learning”. Afterwards, in the second stage, experimental data are evaluated and parameters of “other” kinetic curves are computed without solving anymore the system of differential equations. The combined ED-ANNs approach has been applied to solve several kinetic systems. It was also demonstrated that using ANNs, the optimization of complex chemical systems can be achieved even not knowing or determining the values of the rate constants. Moreover, the solution of differential equations is here not necessary, as well. Using ED the number of experiments can be reduced substantially. Methodology of ED-ANNs applied to multicomponent analysis shows advantages over classical methods while the knowledge of kinetic reactions is not needed. ANNs computation in kinetics is robust as shown evaluating the effect of experimental errors and it is of general applicability. |
Související projekty: |