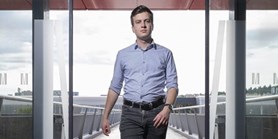
Zde se nacházíte:
Informace o publikaci
Nonparametric estimation of information-based measures of statistical dispersion
Autoři | |
---|---|
Rok publikování | 2012 |
Druh | Článek v odborném periodiku |
Časopis / Zdroj | Entropy |
Fakulta / Pracoviště MU | |
Citace | |
Doi | http://dx.doi.org/10.3390/e14071221 |
Obor | Obecná matematika |
Klíčová slova | statistical dispersion; entropy; Fisher information; nonparametric density estimation |
Popis | We address the problem of non-parametric estimation of the recently proposed measures of statistical dispersion of positive continuous random variables. The measures are based on the concepts of differential entropy and Fisher information and describe the "spread" or "variability" of the random variable from a different point of view than the ubiquitously used concept of standard deviation. The maximum penalized likelihood estimation of the probability density function proposed by Good and Gaskins is applied and a complete methodology of how to estimate the dispersion measures with a single algorithm is presented. We illustrate the approach on three standard statistical models describing neuronal activity. |