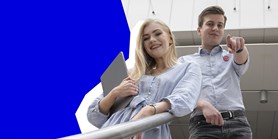
FireProt(DB): database of manually curated protein stability data
Autoři | |
---|---|
Rok publikování | 2021 |
Druh | Článek v odborném periodiku |
Časopis / Zdroj | Nucleic acids research |
Fakulta / Pracoviště MU | |
Citace | |
www | https://academic.oup.com/nar/article/49/D1/D319/5964070 |
Doi | http://dx.doi.org/10.1093/nar/gkaa981 |
Klíčová slova | SEQUENCE; PREDICTION; VARIANTS |
Přiložené soubory | |
Popis | The majority of naturally occurring proteins have evolved to function under mild conditions inside the living organisms. One of the critical obstacles for the use of proteins in biotechnological applications is their insufficient stability at elevated temperatures or in the presence of salts. Since experimental screening for stabilizing mutations is typically laborious and expensive, in silico predictors are often used for narrowing down the mutational landscape. The recent advances in machine learning and artificial intelligence further facilitate the development of such computational tools. However, the accuracy of these predictors strongly depends on the quality and amount of data used for training and testing, which have often been reported as the current bottleneck of the approach. To address this problem, we present a novel database of experimental thermostability data for single-point mutants FireProt(DB). The database combines the published datasets, data extracted manually from the recent literature, and the data collected in our laboratory. Its user interface is designed to facilitate both types of the expected use: (i) the interactive explorations of individual entries on the level of a protein or mutation and (ii) the construction of highly customized and machine learning-friendly datasets using advanced searching and filtering. The database is freely available at https://loschmidt.chemi.muni.cz/fireprotdb. |
Související projekty: |